Sufficient Balance Two, Chapter 2
This post was co-authored by the DFS Lab team.
We recently discussed the concept of the “Frontier Blindspot”: a set of biases we think everyone needs to watch out for, including ourselves. In summary:
“This disconnect between the conditions that drove the growth of the digital economies in high income countries and on-the-ground reality in frontier markets creates what we call the Frontier Blindspot. As Western markets digitized their economies, the world developed intuitions about how technology markets are structured, what successful technology companies look like, and so on. This orthodoxy affects everyone, from founders to investors, driving strategy and informing investment decisions.”
This post is a deep dive into two areas we think are often deeply misunderstood — market size and consumer purchasing power in Africa.
Broadly speaking, there are three ways to make money in tech. You can sell stuff to businesses (e.g. Microsoft, TSMC); you can sell stuff to people (e.g. Amazon, Apple, Alibaba); or you can sell people to businesses via advertising (e.g. Facebook, Google, Tencent). Of course, all these companies do other things — AWS is clearly a B2B service — and many companies touch some combination of all three areas, but these are the three main models.
The fundamental role of venture-backed startups is to explore demand across these three areas. Innovations are infinite; engineers can always create new ways to do things, but which of them will people pay for?
While we don’t purport to know in advance (it is an empirical question after all), that doesn’t mean all innovations are created equal. Having a deeper sense of consumer purchasing power is critical to understanding where opportunities are and how they should be attacked — especially in the latter two areas listed above.
In this post, we are going to look at income and spending power in raw numbers. For a given level of income, how much is likely to be discretionary vs. spent on necessities? How many people are there at each level? And so on. These numbers are not everything: there are factors like psychology, culture, macro-conditions, evolving user needs, etc. that go into technology adoption and product purchase decisions. But ignoring the math can very much lead to delusion. They don’t give an answer, but form a kind of boundary set on what is possible.
The data
The main data source we are going to look at is PovCalNet, a decades old project by the World Bank to compile comparable measures of income and consumption across countries. Using detailed household surveys they do their best to estimate the daily consumption per adult in the household. From this database, we can calculate the income distribution in each country and extrapolate to the region. The data does have its limits and like any data set is going to be off by some unknowable margin, but it’s the best data available (see the Annex at the end for more discussion of the data and its limits).
Note: This is the same data as is behind widely cited pieces like this AFDB analysis and related WSJ piece. Both make the case that there is a large and growing middle class in Africa — comparable to China — a contention we believe paints a distorted picture given China’s middle class is 3–5X richer than Africa’s.
First, what does the big picture look like?
The graph below shows that the vast majority of people in Sub-Saharan Africa live on relatively little, and that the bulk of the economic power is concentrated at the top in the top 10% who consume $5 per day or more. This data is aggregated to the regional level but most countries look roughly similar (with a few exceptions like Botswana and South Africa which have many more people over $10/day). This is also qualitatively similar to more wealthy countries — in that most of the purchasing power is concentrated at the top — but here, the actual income values are much smaller. For example, the threshold to enter the top 1% of consumers in Africa is around $20/day/adult consumption which would still be below the national poverty lines in most of the US and Europe.

Note: The figures here are not — strictly speaking — measures of income but of consumption, per day, per adult. So an adult consuming $5/day would consume roughly 30*$5 = $150/month or $1825/year and generally will make about the same in income unless they produce a lot of goods for home consumption (e.g. farming) or are consuming from savings or paying a lot of taxes (which don’t count as consumption) or other special cases. These figures are PPP adjusted so $5 of consumption is assumed to be the amount of good and services someone could buy in the US with $5 USD (not what one could buy in the local economy with $5 USD). Generally, however, thinking of these figures as roughly equal to income is fairly safe. Learn more about the data in our Annex or at this link: http://iresearch.worldbank.org/PovcalNet/introduction.aspx
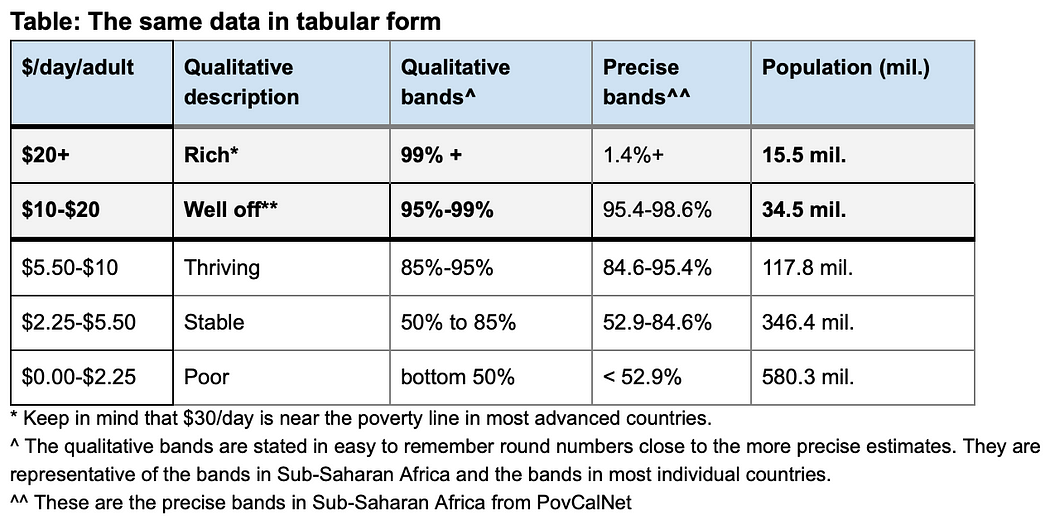
What about the new generation of upwardly mobile, digitally connected, young urban consumers everyone talks about?
Let’s start with the simple question, how many people consume more than $10 a day? This is an arbitrary threshold but we believe most startup product developers target this group (perhaps without knowing what income they are precisely). At this level of income, one starts to find greater smartphone penetration, bank account ownership, and other enablers leading to higher levels of digital sophistication. Tech companies tend to build for these consumers for the fact that they are easier to reach via digital channels, or via in-person marketing in denser cities and because they have more spending power. The data below shows, however, that i) there are relatively few people above this threshold, and ii) they are mostly scattered across 10–15 of the continent’s larger cities. On some level we think this is an easy market to enter, but a challenging one to grow out of. Targeting this milieu alone will likely mean a startup stays niche; they will grow quickly at first as they acquire these wealthier, more digital users, then top out and stall as they hit the limits of the local market. This is one reason why many begin to consider international expansion so early in their lifecycle — to jump to other countries capital cities where there are pockets of higher income digital consumers. We believe many startups in Africa have faced this fate.
Countries sorted by number of people over $10/day.
We have included a few non-African markets for comparison.
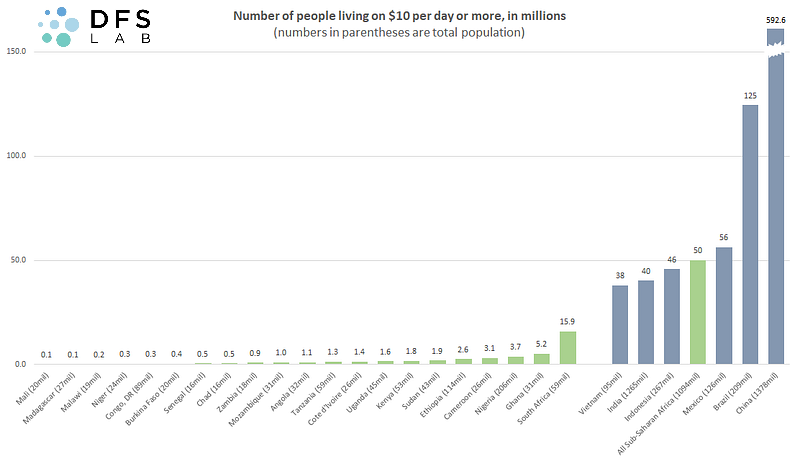
Where is the biggest opportunity?
What if we go further down the income spectrum where there are many more people? Does an increase in the number of people outweigh the loss in income (and the smaller share spent on discretionary expenditure)? Luckily, the data allows us to address this question.
We start by estimating how many people there are at each dollar of income (which we can do with PovCalNet). Then we look at how much discretionary income people are likely to have at each level — this is what they could potentially spend on things other than pure necessities like food, clothing, shelter, and a few other categories. With the important exception of those selling primary necessities or those helping people generate extra income, most B2C tech startups are seeking to make money from people’s discretionary spending. Below is a graph taken from a paper by Banerjee and Duflo (2008) where they quantify the share of income spent on food (the primary necessity good) for people from $1-$10/day and find it goes from 65% at the poorest to 50% at the richer end. To this we add a further 10% to cover clothing, shelter and a few other basics. (This 10% is an estimate but is similar to measurements from other academic research and is likely conservative — i.e. lower than the real figure). From this we calculate a share of discretionary income going from 25% (or $0.25/day) at $1/day to 40% (or $4.00/day) at the higher end. (We assume that at higher income the percentage eventually stabilizes at 70% with very high income but this assumption does not affect the curve shape very much.)
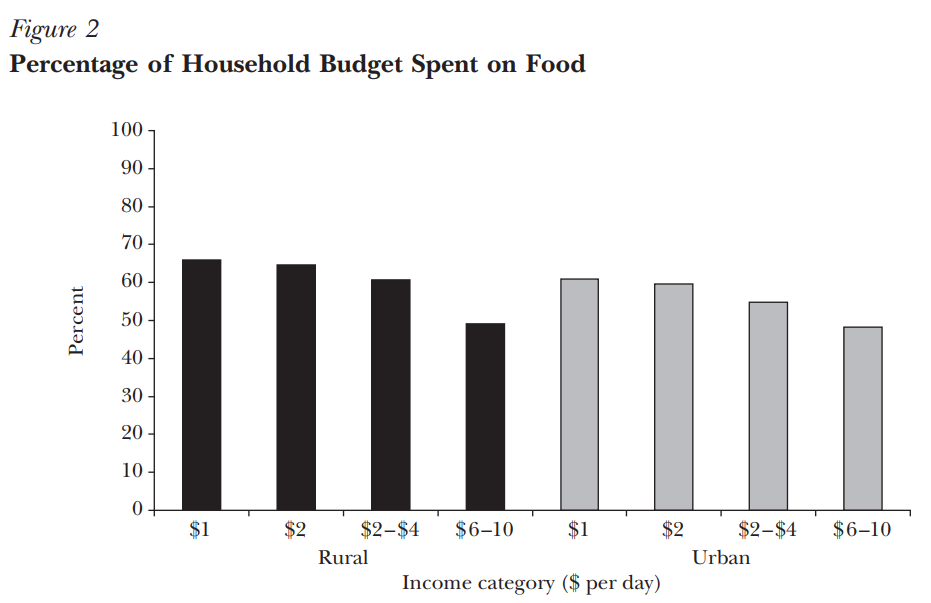
Notes: The PovCalNet interface can do this estimation but takes a lot of work and reformatting so we took the above income banks and fitted a curve to them. Details on this procedure available from the author. Banerjee and Duflo’s (both, recent Nobel Prize winners) paper is here https://economics.mit.edu/files/10881 their numbers are similar to others e.g. http://www.bristol.ac.uk/efm/media/workingpapers/working_papers/pdffiles/dp16677.pdf. They use the same underlying data that powers PovCalNet (the World Bank LSMS surveys) in 13 developing countries (including but not limited to African countries).
Looking at this alongside the number of people at each dollar of income, we can calculate how much aggregate discretionary spending power there is at each income band. As income increases, both income, and the share that is discretionary rise, while the number of people who make that income generally falls. The curve below shows how these tradeoffs play out in the actual data.
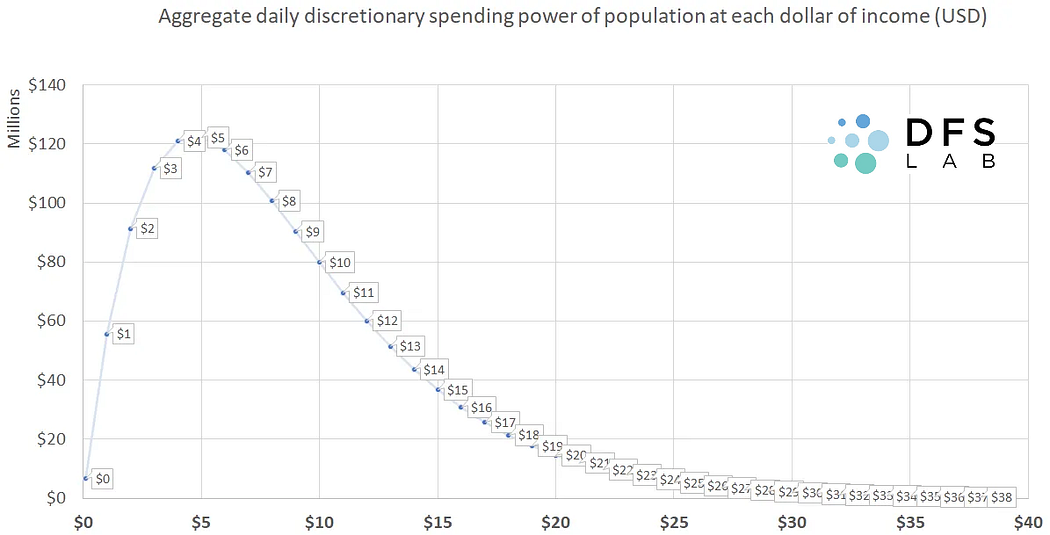
Importantly, we can see that aggregate discretionary spending is relatively small below $3/day despite the fact that the majority of the population live at this level. Their incomes are low and spent almost entirely on necessities. The graph peaks around $4–6 which is where the smaller number of people is best offset by higher income. Beyond that, everyone gets richer rapidly but the population numbers fall off even faster than income goes up — thus absolute spending power falls. Interestingly, the spending power (calculated as the area under the curve) of the consumers in the $5-$10 band of income (which comprise only ~10% of the population) is about double that of the $0-$4/day group (which comprise ~85%). From our experience, these $5-$10 consumers are fairly coherent as a group — i.e. more similar to each other than to $20/day upper income or $2–4/day consumers below them — and thus represent a coherent market segment with significant spending power.
Lowering the cost of acquisition and distribution is key!
But these are just spending figures — startups care about profits, so we have to consider customer acquisition costs as well as the cost of goods sold. Factoring these costs into the equation changes the picture quite a bit. As costs rise, some consumers at the very lowest end will become infeasible (i.e. even if we captured all their discretionary spending, which is nearly impossible, the cost to acquire and serve would still be higher.) Also, the peak of the curve will shift to higher income brackets because, not only is there more money, it’s distributed across fewer people so the cost to acquire a dollar of discretionary spending is assumed lower.
Note: We assume here acquisition costs are pretty similar regardless of income. One might argue that richer customers are more expensive to acquire and serve — we think that the cost of acquiring low income users (less than $5/day) is actually higher than others since it’s often quite hard to reach them over digital channels and requires field force recruiting. These graphs are a simplification since costs will be different for each product and on some level, what entrepreneurs are often doing by “innovating” is discovering less costly ways to serve or acquire users.
The below graph shows net discretionary income after subtracting off cost to acquire and serve each customer for different levels of cost — we can see that the peak goes from $5 to closer to $20 as costs rise and the area under the curve (the total potential market value) shrinks dramatically. Another way to look at this graph is in reverse — any entrepreneur that can design distribution models that lower cost to acquire and serve dramatically will create huge market value and make many more people feasible as customers.
This graph helps illustrate why cost reduction is so important. Initiatives like the Mojaloop open source payment system funded by The Gates Foundation and its partners (Google, PhonePe, Omidyar Network, Rockefeller Foundation and a handful of other charitable and private sector organizations) are one example of initiatives that aim to reduce costs at the industry level.
Net annual spending after subtracting off cost to acquire and serve each customer (USD)
As a proxy for profitability, we calculate the curve for 5 realistic values of annual cost increasing from line 1–5 below
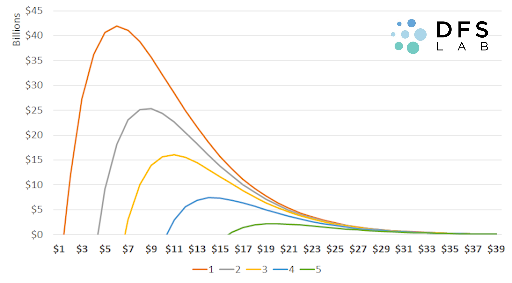
The above analysis assumes that most startups are creating services that target discretionary expenditures. Below we show the same curve when we include all expenditures, including primary necessities. Here we see a much larger market and one where the peak of consumer spending power is now in the $2-$5 range. This comports with the “Bottom of the Pyramid” argument that there is significant spending power among the lower income populations when it comes to food, and other primary necessities. Nevertheless, we still show relatively limited aggregate spending power for the large group of people who live on less than $2/day.
Note: The original BoP article by Prahalad and Hart from 2002 mostly cites examples of primary necessities from the FMCG category like Unilever soap, which is coherent with our analysis. (https://www.strategy-business.com/article/11518?gko=9b3b4)
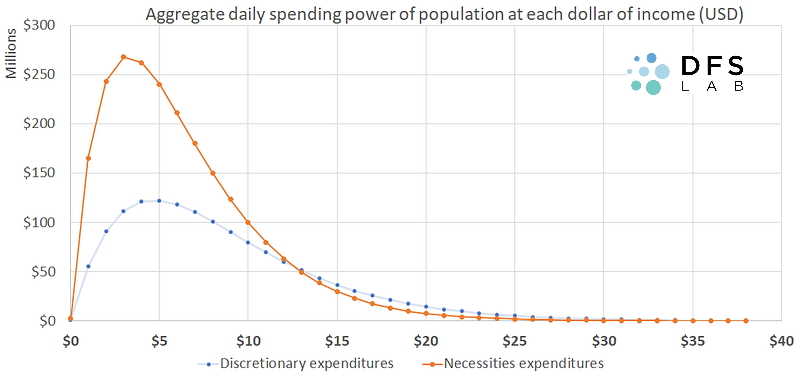
So what do we learn?
The above analysis takes a data driven approach to some very fundamental questions. We see among other observations that:
- Africa is growing fast, has a young and urban population, and many opportunities for improvements in productivity and resource utilization — thus there is much cause for optimism in the tech sector! But purely consumer focused apps will face unique challenges with monetization relative to markets like China, Indonesia, or Latin America.
- Many consumer-focused apps will find easy early traction going after the relatively wealthy $10/day and above segment but will risk a niche business as growth stalls due to the limited size of this population.
- The largest economic opportunity in a pure sense are consumers between $4-$8/day. Here is the largest density of discretionary income due to the capacity to spend beyond simple necessities, and much larger population numbers. That said, costs to acquire and serve these larger numbers of customers will be prohibitive to most and will be the critical factor in success.
- Conversely, those who can create innovative, low cost ways to acquire and serve users in the $4-$8/day category (e.g. partnering with community groups, government, USSD interfaces, viral peer marketing, etc) have the potential to create massive amounts of value by opening these consumers to new markets.
- Also, businesses that focus on primary necessities (by selling directly or improving the value chain efficiency) have a much larger market to target.
- Further, models such as gig work, or seller market places that can dramatically increase people’s income break the logic presented here and could potentially generate large revenue from relatively lower income populations (while improving their income as well, hopefully)
- Finally, while the picture this data paints implies B2C models will face headwinds, we do think that B2B business models that focus on organizing value chains, improving efficiency and resource utilization, digitizing SMEs, and generally increasing productive capacity have huge potential — we will cover this in another piece.
Up Next
Chapter 3: Who is ‘The African Consumer’?: A follow-up to Fortune at the middle of the pyramid
Chapter 4: Physical Ubiquity Redux
Previously
Chapter 1: The Frontier Blindspot